Mathematician Vakhtang Putkaradze receives New Frontiers in Research Funding to make AI more energy efficient
Donna McKinnon - 20 December 2024
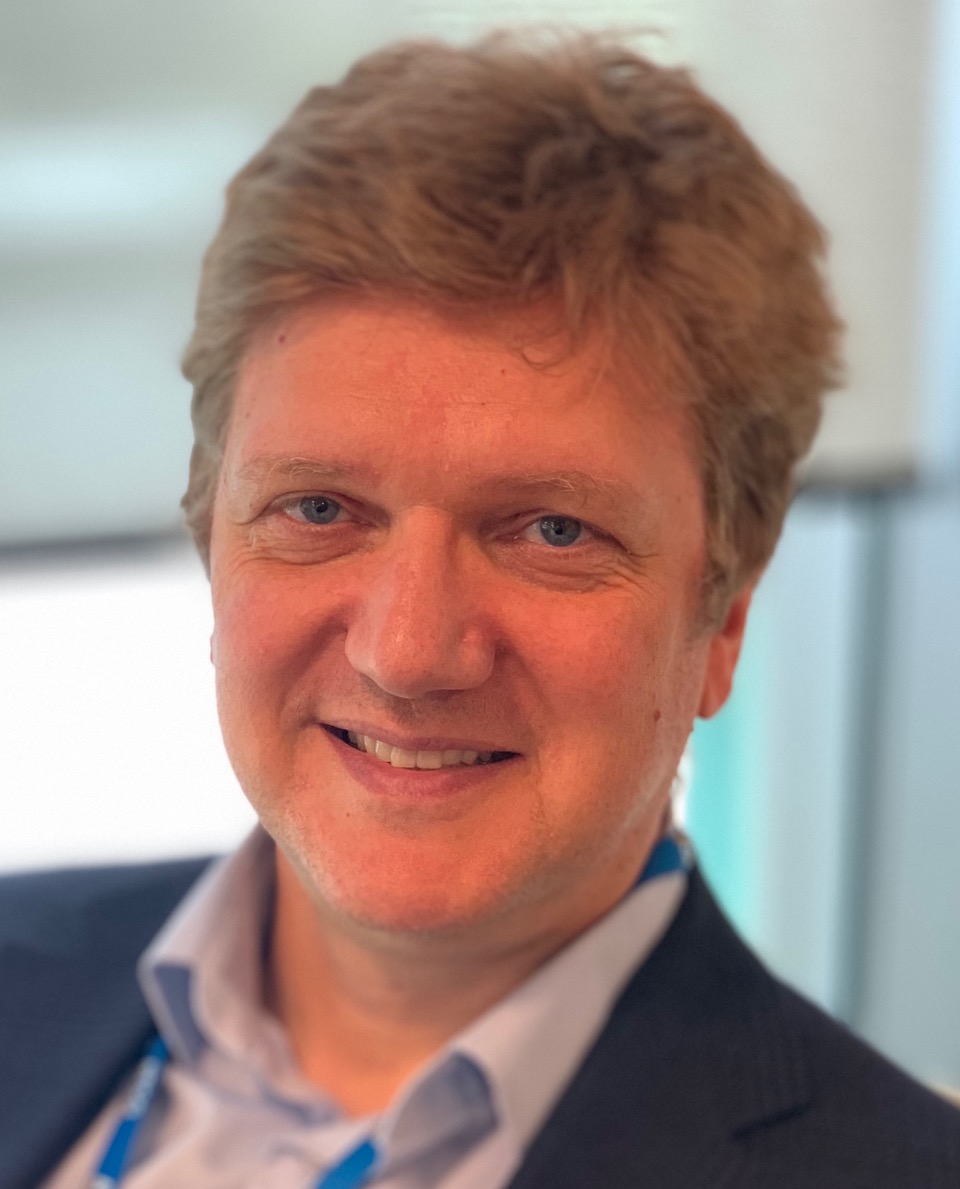
The promise of artificial intelligence to transform our lives and push the boundaries of human achievement has moved from science fiction to an attainable goal — but at what cost? Generative AI like ChatGPT requires a significant amount of energy for training and the generation of human-like responses, but few are aware of its carbon footprint.
“It’s unsustainable,” says Vakhtang Putkaradze, a professor in the Department of Mathematical and Statistical Sciences. “While humanity is trying to remove GHG emissions from areas such as transportation, with deep learning and generative AI, the energy expenditures increase exponentially by an order of magnitude every year or two, likely negating any current efforts in GHG containment. Indeed, making the much bigger model, the GPT-3 with 175 billion parameters, emitted the equivalent of more than 550 tons of CO2 while consuming 1,287 MW hours of electricity (per computer scientist Kate Saenko). It’s the same amount of emissions as a single person taking 550 roundtrip flights between New York and San Francisco.”
The solution, says Putkaradze, may be spiking neural networks (SNNs), which mimic the structure and function of actual human neurons more closely.
“Our neurons activate only when the threshold is reached and thus are much more energy efficient,” he says. “People are now learning from nature in a sense to make new computers that operate like real brains. Traditional computers, underpinning current artificial neural networks, are not very energy efficient; but nature had hundreds of millions of years to optimize the operation of the computers that have evolved.”
Intel, explains Putkaradze, has already been producing the Loihi neuromorphic chip based on the spiking neuron design, which operates on single-watt power, bringing substantial improvements to the energy efficiency of AI applications. The problem is how to program them.
“What we need to do with AI in terms of efficiency is like a factor of a billion, and I don’t see it happening through traditional means,” he says. “What we’re trying to do is train those neuromorphic chips differently, employing ideas borrowed from high-energy physics and string theory and also from chemistry to program SNNs. Although the idea is high risk, the rewards are potentially extremely high.”
Earlier this year, Putkaradze and his team received the New Frontiers in Research Fund from the federal government, which supports world-leading interdisciplinary, international, high-risk / high-reward, transformative and rapid-response Canadian-led research.
His collaborators on the project, Novel methods for energy-efficient machine learning, include fellow mathematicians and co-PI Terry Gannon, Vincent Bouchard, Michael Serpe from the U of A’s Department of Chemistry and Maksim Bazhenov from the University of California, San Diego.
“What we have on the Intel side is equivalent to a dragonfly brain, which is a pretty impressive brain if you consider what a dragonfly can do in terms of the number of connections, something like one million neurons,” says Putkaradze.
“My collaborators are experts in high energy physics — we’re talking about pathways in the brain, but not the human brain; we want to program a small silicon brain. How do you establish those synaptic connections? We also want to establish a network of connections using chemicals and then learn from it for the benefit of the theory. We will at least try to carve out the set of problems for which our methods are applicable. Ideally, you solve everything.”
Putkaradze first started to think about the energy expenditures of AI because of his experiences in industry with ATCO as Vice President of Transformation, Science and Technology. As people were getting excited about generative AI, he wondered: what was supplying the energy? He already knew how difficult it was managing energy supply and consumption, so how would ‘energy hungry’ AI fit into the grid?
If we really want to go in that direction, he says, we need to look at energy efficient methods of reliably programming these computers to do useful things.
“If we solve neuromorphic computers, it could be huge.”
Putkaradze and his colleagues are currently looking to form a neuromorphic computing working group. If you are interested, please contact Professor Putkaradze here.