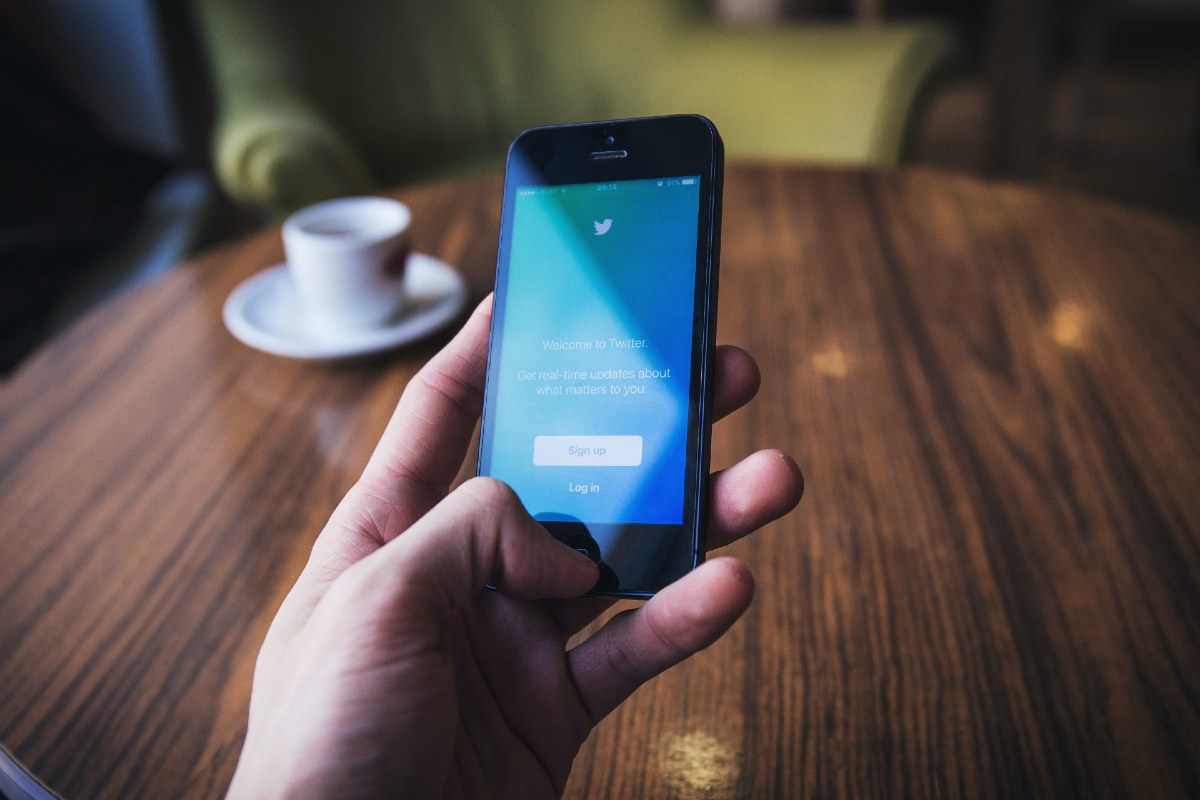
A new machine learning model can detect early signs of depression in written text—including on Twitter—according to a new study by University of Alberta computing scientists. Photo credit: Unsplash
A new machine learning model can detect early signs of depression in written text, according to a new study by University of Alberta computing scientists.
Created by Nawshad Farruque, graduate student in the Department of Computing Science, the model is designed to identify linguistic clues that detect depression in day-to-day communication, such as content on Twitter.
“The outcome of our study is that we can build useful predictive models that can accurately identify depressive language,” said Farruque. “While we are using the model to identify depressive language on Twitter, the model can be easily applied to text from other domains for detecting depression.”
The English-language model was developed using samples of writing by individuals who identify as depressed from online depression forums. The machine learning algorithm is then trained to identify depressive language in tweets. “This is the first study to show that depressive language has a specific linguistic representation,” said Farruque. “We demonstrate that it is possible to identify it, transfer it, and further use it for depressive language detection tasks.”
And there are many potential applications, from detecting the early signs of depression to helping clinicians monitor the effectiveness of treatment for their patients over time.
“Our depressive language detection algorithm could be integrated with a chatbot that talks with seniors and can flag signs of loneliness and depression,” added Farruque. “Another potential application could be to monitor the messages of high school students to identify whether they are suffering from depression.”
Farruque completed this research under the supervision of Osmar Zaïane, professor in the Department of Computing Science and director at the Alberta Machine Intelligence Institute (AMII), and Randy Goebel, professor in the Department of Computing Science and AMII fellow. Drawing from world-leading academic research, including oversight by clinical psychiatrists at the University of Alberta, AMII helps Alberta workers reskill and upskill for high-demand careers in artificial intelligence and guides Alberta-based businesses as they implement artificial intelligence across operations and build their in-house capabilities and teams.
Funding for this research was provided by AMII and the Natural Sciences and Engineering Research Council of Canada (NSERC).
The paper, “Augmenting Semantic Representation of Depressive Language: From Forums to Microblogs,” was published at the European Conference on Machine Learning and Principles and Practice of Knowledge Discovery in Database (doi: 10.1007/978-3-030-46133-1_22).